การขุดข้อมูล นิรุกติศาสตร์และพื้นหลัง
ในทศวรรษที่ 1960 นักสถิติและนักเศรษฐศาสตร์ใช้คำศัพท์เช่นการตกปลาข้อมูลหรือการขุดลอกข้อมูลเพื่ออ้างถึงสิ่งที่พวกเขาคิดว่าการวิเคราะห์ข้อมูลนั้นไม่ดีโดยไม่มีสมมติฐานเบื้องต้น คำว่า "การขุดข้อมูล" ถูกนำไปใช้ในทางที่สำคัญในทำนองเดียวกันโดยนักเศรษฐศาสตร์Michael Lovellในบทความที่ตีพิมพ์ในReview of Economic Studiesในปี 1983 [11] [12] Lovell ระบุว่าการปฏิบัติ "ปลอมตัวภายใต้นามแฝงที่หลากหลาย จาก "การทดลอง" (เชิงบวก) เป็น "การตกปลา" หรือ "การสอดแนม" (เชิงลบ) คำว่าการขุดข้อมูลปรากฏในชุมชนฐานข้อมูลประมาณปี 1990 โดยทั่วไปมีความหมายเชิงบวก ในช่วงเวลาสั้น ๆ ในปี 1980 วลี "การขุดฐานข้อมูล" ™ถูกนำมาใช้ แต่เนื่องจาก HNC ซึ่งเป็น บริษัท ในซานดิเอโกได้รับการจดทะเบียนเครื่องหมายการค้าเพื่อนำเสนอเวิร์คสเตชั่นการขุดฐานข้อมูล [13]นักวิจัยจึงหันไปทำเหมืองข้อมูล เงื่อนไขอื่น ๆ ที่นำมาใช้ ได้แก่โบราณคดีข้อมูล , การเก็บเกี่ยวข้อมูล , การค้นพบข้อมูล , การสกัดความรู้อื่น ๆเกรกอรี่ Piatetsky-ชาปิโรบัญญัติศัพท์คำว่า "ค้นพบความรู้ในฐานข้อมูล" สำหรับการประชุมเชิงปฏิบัติการครั้งแรกในหัวข้อเดียวกัน(KDD-1989)และในระยะนี้กลายเป็นที่นิยมมากขึ้น ในชุมชนAIและการเรียนรู้ของเครื่อง อย่างไรก็ตามคำว่าการขุดข้อมูลได้รับความนิยมมากขึ้นในธุรกิจและชุมชนสื่อมวลชน [14]ปัจจุบันคำว่าการขุดข้อมูลและการค้นพบความรู้ใช้แทนกันได้ ในชุมชนวิชาการเวทีสำคัญสำหรับการวิจัยเริ่มต้นในปี 1995 เมื่อการประชุมนานาชาติครั้งแรกเกี่ยวกับการขุดข้อมูลและการค้นพบความรู้ ( KDD-95 ) เริ่มต้นในมอนทรีออลภายใต้การสนับสนุนของAAAI มีUsama Fayyadและ Ramasamy Uthurusamy เป็นประธานร่วม หนึ่งปีต่อมาในปี 2539 Usama Fayyad ได้เปิดตัววารสารโดย Kluwer ชื่อData Mining and Knowledge Discoveryในฐานะหัวหน้าบรรณาธิการผู้ก่อตั้ง หลังจากนั้นเขาก็เริ่มSIGKDDจดหมายข่าว SIGKDD Explorations [15]การประชุมระหว่างประเทศของ KDD กลายเป็นการประชุมหลักที่มีคุณภาพสูงสุดในการขุดข้อมูลโดยมีอัตราการยอมรับการส่งเอกสารวิจัยต่ำกว่า 18% วารสารData Mining and Knowledge Discoveryเป็นวารสารวิจัยหลักของสาขานี้
พื้นหลัง
การแยกรูปแบบจากข้อมูลด้วยตนเองเกิดขึ้นมาหลายศตวรรษแล้ว วิธีแรก ๆ ในการระบุรูปแบบในข้อมูล ได้แก่ทฤษฎีบทของ Bayes (1700) และการวิเคราะห์การถดถอย (1800) การแพร่หลายความแพร่หลายและพลังที่เพิ่มขึ้นของเทคโนโลยีคอมพิวเตอร์ทำให้ความสามารถในการรวบรวมการจัดเก็บและการจัดการข้อมูลเพิ่มขึ้นอย่างมาก ในฐานะที่เป็นชุดข้อมูลที่มีการเติบโตในขนาดและความซับซ้อนโดยตรง "มือบน" การวิเคราะห์ข้อมูลได้มากขึ้นรับผลดีกับทางอ้อมการประมวลผลข้อมูลอัตโนมัติรับความช่วยเหลือจากการค้นพบอื่น ๆ ในวิทยาการคอมพิวเตอร์เป็นพิเศษในด้านการเรียนรู้ของเครื่องเช่นเครือข่ายประสาท , การวิเคราะห์กลุ่ม , ขั้นตอนวิธีพันธุกรรม (1950) ต้นไม้ตัดสินใจและกฎการตัดสินใจ (1960) และเครื่องสนับสนุนเวกเตอร์ (1990) การขุดข้อมูลเป็นกระบวนการของการใช้วิธีการเหล่านี้โดยมีจุดประสงค์เพื่อเปิดเผยรูปแบบที่ซ่อนอยู่ [16]ในชุดข้อมูลขนาดใหญ่ มันเชื่อมช่องว่างจากสถิติประยุกต์และปัญญาประดิษฐ์ (ซึ่งโดยปกติจะให้พื้นหลังทางคณิตศาสตร์) กับการจัดการฐานข้อมูลโดยใช้ประโยชน์จากวิธีการจัดเก็บและจัดทำดัชนีข้อมูลในฐานข้อมูลเพื่อดำเนินการอัลกอริธึมการเรียนรู้และการค้นพบที่แท้จริงได้อย่างมีประสิทธิภาพมากขึ้นทำให้สามารถนำวิธีการดังกล่าวไปใช้กับ ชุดข้อมูลที่ใหญ่กว่าเดิม
กระบวนการ
The knowledge discovery in databases (KDD) process is commonly defined with the stages: Selection Pre-processing Transformation Data mining Interpretation/evaluation.[5] It exists, however, in many variations on this theme, such as the Cross-industry standard process for data mining (CRISP-DM) which defines six phases: Business understanding Data understanding Data preparation Modeling Evaluation Deployment or a simplified process such as (1) Pre-processing, (2) Data Mining, and (3) Results Validation. Polls conducted in 2002, 2004, 2007 and 2014 show that the CRISP-DM methodology is the leading methodology used by data miners.[17] The only other data mining standard named in these polls was SEMMA. However, 3–4 times as many people reported using CRISP-DM. Several teams of researchers have published reviews of data mining process models,[18] and Azevedo and Santos conducted a comparison of CRISP-DM and SEMMA in 2008.[19] Pre-processing Before data mining algorithms can be used, a target data set must be assembled. As data mining can only uncover patterns actually present in the data, the target data set must be large enough to contain these patterns while remaining concise enough to be mined within an acceptable time limit. A common source for data is a data mart or data warehouse. Pre-processing is essential to analyze the multivariate data sets before data mining. The target set is then cleaned. Data cleaning removes the observations containing noise and those with missing data. Data mining Data mining involves six common classes of tasks:[5] Anomaly detection (outlier/change/deviation detection) – The identification of unusual data records, that might be interesting or data errors that require further investigation.
Association rule learning (dependency modeling) – Searches for relationships between variables. For example, a supermarket might gather data on customer purchasing habits. Using association rule learning, the supermarket can determine which products are frequently bought together and use this information for marketing purposes. This is sometimes referred to as market basket analysis.
Clustering – is the task of discovering groups and structures in the data that are in some way or another "similar", without using known structures in the data.
Classification – is the task of generalizing known structure to apply to new data. For example, an e-mail program might attempt to classify an e-mail as "legitimate" or as "spam".
Regression – attempts to find a function that models the data with the least error that is, for estimating the relationships among data or datasets.
Summarization – providing a more compact representation of the data set, including visualization and report generation. Results validation An example of data produced by data dredging through a bot operated by statistician Tyler Vigen, apparently showing a close link between the best word winning a spelling bee competition and the number of people in the United States killed by venomous spiders. The similarity in trends is obviously a coincidence. An example of data produced by data dredging through a bot operated by statistician Tyler Vigen, apparently showing a close link between the best word winning a spelling bee competition and the number of people in the United States killed by venomous spiders. The similarity in trends is obviously a coincidence. Data mining can unintentionally be misused, and can then produce results that appear to be significant; but which do not actually predict future behavior and cannot be reproduced on a new sample of data and bear little use. Often this results from investigating too many hypotheses and not performing proper statistical hypothesis testing. A simple version of this problem in machine learning is known as overfitting, but the same problem can arise at different phases of the process and thus a train/test split—when applicable at all—may not be sufficient to prevent this from happening.[20] The final step of knowledge discovery from data is to verify that the patterns produced by the data mining algorithms occur in the wider data set. Not all patterns found by data mining algorithms are necessarily valid. It is common for data mining algorithms to find patterns in the training set which are not present in the general data set. This is called overfitting. To overcome this, the evaluation uses a test set of data on which the data mining algorithm was not trained. The learned patterns are applied to this test set, and the resulting output is compared to the desired output. For example, a data mining algorithm trying to distinguish "spam" from "legitimate" emails would be trained on a training set of sample e-mails. Once trained, the learned patterns would be applied to the test set of e-mails on which it had not been trained. The accuracy of the patterns can then be measured from how many e-mails they correctly classify. Several statistical methods may be used to evaluate the algorithm, such as ROC curves. If the learned patterns do not meet the desired standards, subsequently it is necessary to re-evaluate and change the pre-processing and data mining steps. If the learned patterns do meet the desired standards, then the final step is to interpret the learned patterns and turn them into knowledge.
การวิจัย
The premier professional body in the field is the Association for Computing Machinery's (ACM) Special Interest Group (SIG) on Knowledge Discovery and Data Mining (SIGKDD).[21][22] Since 1989, this ACM SIG has hosted an annual international conference and published its proceedings,[23] and since 1999 it has published a biannual academic journal titled "SIGKDD Explorations".[24] Computer science conferences on data mining include: CIKM Conference – ACM Conference on Information and Knowledge Management
European Conference on Machine Learning and Principles and Practice of Knowledge Discovery in Databases
KDD Conference – ACM SIGKDD Conference on Knowledge Discovery and Data Mining Data mining topics are also present on many data management/database conferences such as the ICDE Conference, SIGMOD Conference and International Conference on Very Large Data Bases
มาตรฐาน
There have been some efforts to define standards for the data mining process, for example, the 1999 European Cross Industry Standard Process for Data Mining (CRISP-DM 1.0) and the 2004 Java Data Mining standard (JDM 1.0). Development on successors to these processes (CRISP-DM 2.0 and JDM 2.0) was active in 2006 but has stalled since. JDM 2.0 was withdrawn without reaching a final draft. For exchanging the extracted models—in particular for use in predictive analytics—the key standard is the Predictive Model Markup Language (PMML), which is an XML-based language developed by the Data Mining Group (DMG) and supported as exchange format by many data mining applications. As the name suggests, it only covers prediction models, a particular data mining task of high importance to business applications. However, extensions to cover (for example) subspace clustering have been proposed independently of the DMG.[25]
การใช้งานที่โดดเด่น
Data mining is used wherever there is digital data available today. Notable examples of data mining can be found throughout business, medicine, science, and surveillance.
ข้อกังวลเกี่ยวกับความเป็นส่วนตัวและจริยธรรม
While the term "data mining" itself may have no ethical implications, it is often associated with the mining of information in relation to peoples' behavior (ethical and otherwise).[26] The ways in which data mining can be used can in some cases and contexts raise questions regarding privacy, legality, and ethics.[27] In particular, data mining government or commercial data sets for national security or law enforcement purposes, such as in the Total Information Awareness Program or in ADVISE, has raised privacy concerns.[28][29] Data mining requires data preparation which uncovers information or patterns which compromise confidentiality and privacy obligations. A common way for this to occur is through data aggregation. Data aggregation involves combining data together (possibly from various sources) in a way that facilitates analysis (but that also might make identification of private, individual-level data deducible or otherwise apparent).[30] This is not data mining per se, but a result of the preparation of data before—and for the purposes of—the analysis. The threat to an individual's privacy comes into play when the data, once compiled, cause the data miner, or anyone who has access to the newly compiled data set, to be able to identify specific individuals, especially when the data were originally anonymous.[31][32][33] It is recommended[according to whom?] to be aware of the following before data are collected:[30] The purpose of the data collection and any (known) data mining projects;
How the data will be used;
Who will be able to mine the data and use the data and their derivatives;
The status of security surrounding access to the data;
How collected data can be updated. Data may also be modified so as to become anonymous, so that individuals may not readily be identified.[30] However, even "anonymized" data sets can potentially contain enough information to allow identification of individuals, as occurred when journalists were able to find several individuals based on a set of search histories that were inadvertently released by AOL.[34] The inadvertent revelation of personally identifiable information leading to the provider violates Fair Information Practices. This indiscretion can cause financial, emotional, or bodily harm to the indicated individual. In one instance of privacy violation, the patrons of Walgreens filed a lawsuit against the company in 2011 for selling prescription information to data mining companies who in turn provided the data to pharmaceutical companies.[35] Situation in Europe Europe has rather strong privacy laws, and efforts are underway to further strengthen the rights of the consumers. However, the U.S.–E.U. Safe Harbor Principles, developed between 1998 and 2000, currently effectively expose European users to privacy exploitation by U.S. companies. As a consequence of Edward Snowden's global surveillance disclosure, there has been increased discussion to revoke this agreement, as in particular the data will be fully exposed to the National Security Agency, and attempts to reach an agreement with the United States have failed.[36] In the United Kingdom in particular there have been cases of corporations using data mining as a way to target certain groups of customers forcing them to pay unfairly high prices. These groups tend to be people of lower socio-economic status who are not savvy to the ways they can be exploited in digital market places.[37] Situation in the United States In the United States, privacy concerns have been addressed by the US Congress via the passage of regulatory controls such as the Health Insurance Portability and Accountability Act (HIPAA). The HIPAA requires individuals to give their "informed consent" regarding information they provide and its intended present and future uses. According to an article in Biotech Business Week, "'[i]n practice, HIPAA may not offer any greater protection than the longstanding regulations in the research arena,' says the AAHC. More importantly, the rule's goal of protection through informed consent is approach a level of incomprehensibility to average individuals."[38] This underscores the necessity for data anonymity in data aggregation and mining practices. U.S. information privacy legislation such as HIPAA and the Family Educational Rights and Privacy Act (FERPA) applies only to the specific areas that each such law addresses. The use of data mining by the majority of businesses in the U.S. is not controlled by any legislation.
กฎหมายลิขสิทธิ์
Situation in Europe Under European copyright and database laws, the mining of in-copyright works (such as by web mining) without the permission of the copyright owner is not legal. Where a database is pure data in Europe, it may be that there is no copyright—but database rights may exist so data mining becomes subject to intellectual property owners' rights that are protected by the Database Directive. On the recommendation of the Hargreaves review, this led to the UK government to amend its copyright law in 2014 to allow content mining as a limitation and exception.[39] The UK was the second country in the world to do so after Japan, which introduced an exception in 2009 for data mining. However, due to the restriction of the Information Society Directive (2001), the UK exception only allows content mining for non-commercial purposes. UK copyright law also does not allow this provision to be overridden by contractual terms and conditions. The European Commission facilitated stakeholder discussion on text and data mining in 2013, under the title of Licences for Europe.[40] The focus on the solution to this legal issue, such as licensing rather than limitations and exceptions, led to representatives of universities, researchers, libraries, civil society groups and open access publishers to leave the stakeholder dialogue in May 2013.[41] Situation in the United States US copyright law, and in particular its provision for fair use, upholds the legality of content mining in America, and other fair use countries such as Israel, Taiwan and South Korea. As content mining is transformative, that is it does not supplant the original work, it is viewed as being lawful under fair use. For example, as part of the Google Book settlement the presiding judge on the case ruled that Google's digitization project of in-copyright books was lawful, in part because of the transformative uses that the digitization project displayed—one being text and data mining.[42]
ซอฟต์แวร์
Free open-source data mining software and applications The following applications are available under free/open-source licenses. Public access to application source code is also available. Carrot2: Text and search results clustering framework.
A chemical structure miner and web search engine.
ELKI: A university research project with advanced cluster analysis and outlier detection methods written in the Java language.
GATE: a natural language processing and language engineering tool.
KNIME: The Konstanz Information Miner, a user-friendly and comprehensive data analytics framework.
Massive Online Analysis (MOA): a real-time big data stream mining with concept drift tool in the Java programming language.
MEPX - cross-platform tool for regression and classification problems based on a Genetic Programming variant.
ML-Flex: A software package that enables users to integrate with third-party machine-learning packages written in any programming language, execute classification analyses in parallel across multiple computing nodes, and produce HTML reports of classification results.
mlpack: a collection of ready-to-use machine learning algorithms written in the C++ language.
NLTK (Natural Language Toolkit): A suite of libraries and programs for symbolic and statistical natural language processing (NLP) for the Python language.
OpenNN: Open neural networks library.
Orange: A component-based data mining and machine learning software suite written in the Python language.
PSPP: Data mining and statistics software under the GNU Project similar to SPSS
R: A programming language and software environment for statistical computing, data mining, and graphics. It is part of the GNU Project.
scikit-learn is an open-source machine learning library for the Python programming language
Torch: An open-source deep learning library for the Lua programming language and scientific computing framework with wide support for machine learning algorithms.
UIMA: The UIMA (Unstructured Information Management Architecture) is a component framework for analyzing unstructured content such as text, audio and video – originally developed by IBM.
Weka: A suite of machine learning software applications written in the Java programming language. Proprietary data-mining software and applications The following applications are available under proprietary licenses. Angoss KnowledgeSTUDIO: data mining tool
LIONsolver: an integrated software application for data mining, business intelligence, and modeling that implements the Learning and Intelligent OptimizatioN (LION) approach.
Megaputer Intelligence: data and text mining software is called PolyAnalyst.
Microsoft Analysis Services: data mining software provided by Microsoft.
NetOwl: suite of multilingual text and entity analytics products that enable data mining.
Oracle Data Mining: data mining software by Oracle Corporation.
PSeven: platform for automation of engineering simulation and analysis, multidisciplinary optimization and data mining provided by DATADVANCE.
Qlucore Omics Explorer: data mining software.
RapidMiner: An environment for machine learning and data mining experiments.
SAS Enterprise Miner: data mining software provided by the SAS Institute.
SPSS Modeler: data mining software provided by IBM.
STATISTICA Data Miner: data mining software provided by StatSoft.
Tanagra: Visualisation-oriented data mining software, also for teaching.
Vertica: data mining software provided by Hewlett-Packard.
Google Cloud Platform: automated custom ML models managed by Google.
Amazon SageMaker: managed service provided by Amazon for creating & productionising custom ML models.
ดูสิ่งนี้ด้วย
Methods Agent mining
Anomaly/outlier/change detection
Association rule learning
Bayesian networks
Classification
Cluster analysis
Decision trees
Ensemble learning
Factor analysis
Genetic algorithms
Intention mining
Learning classifier system
Multilinear subspace learning
Neural networks
Regression analysis
Sequence mining
Structured data analysis
Support vector machines
Text mining
Time series analysis Application domains Analytics
Behavior informatics
Big data
Bioinformatics
Business intelligence
Data analysis
Data warehouse
Decision support system
Domain driven data mining
Drug discovery
Exploratory data analysis
Predictive analytics
Web mining Application examples Automatic number plate recognition in the United Kingdom
Customer analytics
Educational data mining
National Security Agency
Quantitative structure–activity relationship
Surveillance / Mass surveillance (e.g., Stellar Wind) Related topics For more information about extracting information out of data (as opposed to analyzing data) , see: Data integration
Data transformation
Electronic discovery
Information extraction
Information integration
Named-entity recognition
Profiling (information science)
Psychometrics
Social media mining
Surveillance capitalism
Web scraping Other resources International Journal of Data Warehousing and Mining
อ้างอิง
^ a b c "Data Mining Curriculum". ACM SIGKDD. 2006-04-30 . ^ Clifton, Christopher (2010). "Encyclopædia Britannica: Definition of Data Mining" . ^ Hastie, Trevor; Tibshirani, Robert; Friedman, Jerome (2009). "The Elements of Statistical Learning: Data Mining, Inference, and Prediction". Archived from the original on 2009-11-10 . ^ Han, Kamber, Pei, Jaiwei, Micheline, Jian (2011). Data Mining: Concepts and Techniques (3rd ed.). Morgan Kaufmann. ISBN 978-0-12-381479-1 . ^ a b c Fayyad, Usama; Piatetsky-Shapiro, Gregory; Smyth, Padhraic (1996). "From Data Mining to Knowledge Discovery in Databases" (PDF) . ^ Han, Jiawei; Kamber, Micheline (2001). Data mining: concepts and techniques. Morgan Kaufmann. p. 5. ISBN 978-1-55860-489-6 . Thus, data mining should have been more appropriately named "knowledge mining from data," which is unfortunately somewhat long ^ OKAIRP 2005 Fall Conference, Arizona State University Archived 2014-02-01 at the Wayback Machine ^ Witten, Ian H.; Frank, Eibe; Hall, Mark A. (2011). Data Mining: Practical Machine Learning Tools and Techniques (3 ed.). Elsevier. ISBN 978-0-12-374856-0 . ^ Bouckaert, Remco R.; Frank, Eibe; Hall, Mark A.; Holmes, Geoffrey; Pfahringer, Bernhard; Reutemann, Peter; Witten, Ian H. (2010). "WEKA Experiences with a Java open-source project". Journal of Machine Learning Research. 11: 2533–2541. the original title, "Practical machine learning", was changed ... The term "data mining" was [added] primarily for marketing reasons. ^ Olson, D. L. (2007). Data mining in business services. Service Business, 1(3), 181–193. doi:10.1007/s11628-006-0014-7 ^ Lovell, Michael C. (1983). "Data Mining". The Review of Economics and Statistics. 65 (1): 1–12. doi:10.2307/1924403. JSTOR 1924403. ^ Charemza, Wojciech W.; Deadman, Derek F. (1992). "Data Mining". New Directions in Econometric Practice. Aldershot: Edward Elgar. pp. 14–31. ISBN 1-85278-461-X . ^ Mena, Jesús (2011). Machine Learning Forensics for Law Enforcement, Security, and Intelligence. Boca Raton, FL: CRC Press (Taylor & Francis Group). ISBN 978-1-4398-6069-4 . ^ Piatetsky-Shapiro, Gregory; Parker, Gary (2011). "Lesson: Data Mining, and Knowledge Discovery: An Introduction". Introduction to Data Mining. KD Nuggets . ^ Fayyad, Usama (15 June 1999). "First Editorial by Editor-in-Chief". SIGKDD Explorations. 13 (1): 102. doi:10.1145/2207243.2207269. S2CID 13314420 . ^ Kantardzic, Mehmed (2003). Data Mining: Concepts, Models, Methods, and Algorithms . John Wiley & Sons. ISBN 978-0-471-22852-3 . OCLC 50055336. ^ Gregory Piatetsky-Shapiro (2002) KDnuggets Methodology Poll, Gregory Piatetsky-Shapiro (2004) KDnuggets Methodology Poll, Gregory Piatetsky-Shapiro (2007) KDnuggets Methodology Poll, Gregory Piatetsky-Shapiro (2014) KDnuggets Methodology Poll ^ Lukasz Kurgan and Petr Musilek: "A survey of Knowledge Discovery and Data Mining process models". The Knowledge Engineering Review. Volume 21 Issue 1, March 2006, pp 1–24, Cambridge University Press, New York, doi:10.1017/S0269888906000737 ^ Azevedo, A. and Santos, M. F. KDD, SEMMA and CRISP-DM: a parallel overview Archived 2013-01-09 at the Wayback Machine. In Proceedings of the IADIS European Conference on Data Mining 2008, pp 182–185. ^ Hawkins, Douglas M (2004). "The problem of overfitting". Journal of Chemical Information and Computer Sciences. 44 (1): 1–12. doi:10.1021/ci0342472. PMID 14741005. ^ "Microsoft Academic Search: Top conferences in data mining". Microsoft Academic Search. ^ "Google Scholar: Top publications - Data Mining & Analysis". Google Scholar. ^ Proceedings Archived 2010-04-30 at the Wayback Machine, International Conferences on Knowledge Discovery and Data Mining, ACM, New York. ^ SIGKDD Explorations, ACM, New York. ^ Günnemann, Stephan; Kremer, Hardy; Seidl, Thomas (2011). "An extension of the PMML standard to subspace clustering models". Proceedings of the 2011 workshop on Predictive markup language modeling. p. 48. doi:10.1145/2023598.2023605. ISBN 978-1-4503-0837-3 . S2CID 14967969. ^ Seltzer, William (2005). "The Promise and Pitfalls of Data Mining: Ethical Issues" (PDF) . ASA Section on Government Statistics. American Statistical Association. ^ Pitts, Chip (15 March 2007). "The End of Illegal Domestic Spying? Don't Count on It". Washington Spectator. Archived from the original on 2007-11-28. ^ Taipale, Kim A. (15 December 2003). "Data Mining and Domestic Security: Connecting the Dots to Make Sense of Data". Columbia Science and Technology Law Review. 5 (2). OCLC 45263753. SSRN 546782 . ^ Resig, John. "A Framework for Mining Instant Messaging Services" (PDF) . ^ a b c Think Before You Dig: Privacy Implications of Data Mining & Aggregation Archived 2008-12-17 at the Wayback Machine, NASCIO Research Brief, September 2004 ^ Ohm, Paul. "Don't Build a Database of Ruin". Harvard Business Review. ^ Darwin Bond-Graham, Iron Cagebook – The Logical End of Facebook's Patents, 2013.12.03 ^ Darwin Bond-Graham, Inside the Tech industry's Startup Conference, 2013.09.11 ^ AOL search data identified individuals, SecurityFocus, August 2006 ^ Kshetri, Nir (2014). "Big data׳s impact on privacy, security and consumer welfare" (PDF) . Telecommunications Policy. 38 (11): 1134–1145. ^ Weiss, Martin A.; Archick, Kristin (19 May 2016). "U.S.–E.U. Data Privacy: From Safe Harbor to Privacy Shield" (PDF) . Washington, D.C. Congressional Research Service. p. 6. R44257 . On October 6, 2015, the CJEU ... issued a decision that invalidated Safe Harbor (effective immediately), as currently implemented. ^ Parker, George. “UK Companies Targeted for Using Big Data to Exploit Customers.” Subscribe to Read | Financial Times, Financial Times, 30 Sept. 2018, ^ Biotech Business Week Editors (June 30, 2008); BIOMEDICINE; HIPAA Privacy Rule Impedes Biomedical Research, Biotech Business Week, retrieved 17 November 2009 from LexisNexis Academic ^ UK Researchers Given Data Mining Right Under New UK Copyright Laws. Archived June 9, 2014, at the Wayback Machine Retrieved 14 November 2014 ^ "Licences for Europe – Structured Stakeholder Dialogue 2013". European Commission . ^ "Text and Data Mining:Its importance and the need for change in Europe". Association of European Research Libraries . ^ "Judge grants summary judgment in favor of Google Books – a fair use victory". Antonelli Law Ltd .
อ่านเพิ่มเติม
Cabena, Peter; Hadjnian, Pablo; Stadler, Rolf; Verhees, Jaap; Zanasi, Alessandro (1997); Discovering Data Mining: From Concept to Implementation , Prentice Hall, ISBN 0-13-743980-6
, Prentice Hall, ISBN 0-13-743980-6 M.S. Chen, J. Han, P.S. Yu (1996) "Data mining: an overview from a database perspective". Knowledge and data Engineering, IEEE Transactions on 8 (6), 866–883
on 8 (6), 866–883 Feldman, Ronen; Sanger, James (2007); The Text Mining Handbook , Cambridge University Press, ISBN 978-0-521-83657-9
, Cambridge University Press, ISBN 978-0-521-83657-9 Guo, Yike; and Grossman, Robert (editors) (1999); High Performance Data Mining: Scaling Algorithms, Applications and Systems , Kluwer Academic Publishers
, Kluwer Academic Publishers Han, Jiawei, Micheline Kamber, and Jian Pei. Data mining: concepts and techniques . Morgan kaufmann, 2006.
. Morgan kaufmann, 2006. Hastie, Trevor, Tibshirani, Robert and Friedman, Jerome (2001); The Elements of Statistical Learning: Data Mining, Inference, and Prediction , Springer, ISBN 0-387-95284-5
, Springer, ISBN 0-387-95284-5 Liu, Bing (2007, 2011); Web Data Mining: Exploring Hyperlinks, Contents and Usage Data , Springer, ISBN 3-540-37881-2
, Springer, ISBN 3-540-37881-2 Murphy, Chris (16 May 2011). "Is Data Mining Free Speech?". InformationWeek : 12.
Nisbet, Robert; Elder, John; Miner, Gary (2009); Handbook of Statistical Analysis & Data Mining Applications , Academic Press/Elsevier, ISBN 978-0-12-374765-5
, Academic Press/Elsevier, ISBN 978-0-12-374765-5 Poncelet, Pascal; Masseglia, Florent; and Teisseire, Maguelonne (editors) (October 2007); "Data Mining Patterns: New Methods and Applications", Information Science Reference , ISBN 978-1-59904-162-9
, ISBN 978-1-59904-162-9 Tan, Pang-Ning; Steinbach, Michael; and Kumar, Vipin (2005); Introduction to Data Mining , ISBN 0-321-32136-7
, ISBN 0-321-32136-7 Theodoridis, Sergios; and Koutroumbas, Konstantinos (2009); Pattern Recognition , 4th Edition, Academic Press, ISBN 978-1-59749-272-0
, 4th Edition, Academic Press, ISBN 978-1-59749-272-0 Weiss, Sholom M.; and Indurkhya, Nitin (1998); Predictive Data Mining , Morgan Kaufmann
, Morgan Kaufmann Witten, Ian H.; Frank, Eibe; Hall, Mark A. (30 January 2011). Data Mining: Practical Machine Learning Tools and Techniques (3 ed.). Elsevier. ISBN 978-0-12-374856-0 . (See also Free Weka software)
(See also Free Weka software) Ye, Nong (2003); The Handbook of Data Mining, Mahwah, NJ: Lawrence Erlbaum
4 เทคนิคการสร้างรายการ และ การขุดข้อมูลสำหรับสตาร์ทอัพ
4 เทคนิคการสร้างรายการและ การขุดข้อมูลสำหรับสตาร์ทอัพ ความรู้คือพลัง. ธุรกิจขนาดเล็กและ บริษัท ที่เพิ่งเริ่มต้นต้องใช้ความคิดนี้เมื่อพูดถึงข้อมูลของพวกเขาซึ่งพวกเขาจำเป็นต้องถือเป็นขุมทรัพย์แห่งความรู้ที่แท้จริงที่รอการสำรวจ นั่นคือสิ่งที่เทคนิคการขุดข้อมูลเข้ามา
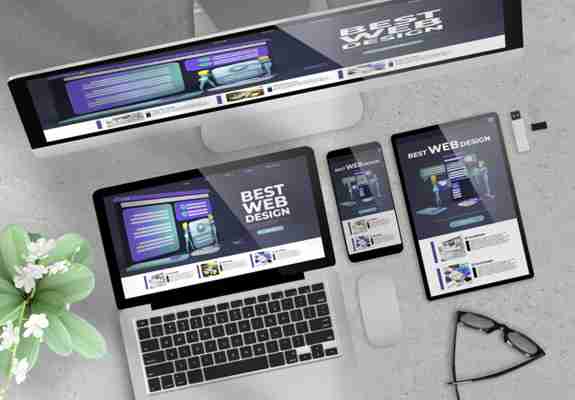
แม้ว่า บริษัท ขนาดเล็กจะเผชิญกับความท้าทายในการใช้ข้อมูลของพวกเขา แต่ก็ไม่ควรถูกขัดขวางจากการใช้เทคนิคที่สามารถช่วยให้พวกเขาได้รับข้อมูลเชิงลึกจากศักยภาพของข้อมูลที่ยังไม่ได้ใช้
สตาร์ทอัพและธุรกิจขนาดเล็กจำเป็นต้องได้รับอำนาจในการปฏิบัติต่อข้อมูลในฐานะสินค้าที่มีคุณค่าและสร้างกลยุทธ์ที่สามารถเปลี่ยนข้อมูลดิบให้กลายเป็นข้อมูลเชิงลึกที่มีคุณค่าซึ่งกระตุ้นการตัดสินใจกลยุทธ์และการเติบโต
การสำรวจข้อมูลที่นำเสนอเกิดขึ้นผ่านการขุดข้อมูลซึ่งเป็นกระบวนการที่ดึงข้อมูลที่มีความหมายและใช้งานได้จากชุดข้อมูลดิบที่มีจำนวนมากขึ้น ตอนนี้ทุกคนคงเคยได้ยินเกี่ยวกับการขุดข้อมูล แต่เทคนิคการขุดข้อมูลที่แตกต่างกันมีหลายอย่างที่จะนำเสนอการเริ่มต้น
เช่นเดียวกับธุรกิจและองค์กรขนาดใหญ่สตาร์ทอัพควรให้ความสำคัญกับเทคนิคการขุดข้อมูลเพื่อสร้างกลยุทธ์สำหรับความสัมพันธ์กับลูกค้าการติดต่ออย่างมืออาชีพและการขายและการตลาด การขุดข้อมูลยังเป็นวิธีการสำหรับสตาร์ทอัพในการตอบคำถามด้วยวิธีการแก้ปัญหาได้รับความสามารถในการจัดการที่ดีขึ้นและจัดสรรทรัพยากรประเมินความเสี่ยงและโอกาสและเตรียมพร้อมสำหรับความท้าทายที่จะเกิดขึ้น
การขุดข้อมูลคืออะไร
เทคนิคการขุดข้อมูลช่วยให้สามารถระบุรูปแบบข้อมูลภายในปริมาณข้อมูลที่มากขึ้นโดยใช้ซอฟต์แวร์และเครื่องมือเฉพาะ และด้วยกระบวนการนี้สตาร์ทอัพสามารถค้นพบข้อมูลเชิงลึกและรับความรู้เกี่ยวกับลูกค้าและการขายและเรียนรู้เพิ่มเติมเกี่ยวกับข้อมูลของพวกเขาและนำข้อมูลเชิงลึกนี้ไปใช้ในวัตถุประสงค์ที่มุ่งเน้นไปที่การพัฒนาประสบการณ์ของลูกค้าการขายและการตลาดกลยุทธ์ทางการตลาดและการตัดสินใจด้านอื่น ๆ ทำให้ทุกธุรกิจต้องเผชิญในชีวิตประจำวัน
เป็นวิธีการใช้ประโยชน์จากข้อมูลดิบในลักษณะที่ทำให้มีจุดมุ่งหมายและมีความหมาย การขุดข้อมูลมีองค์ประกอบของการรวบรวมข้อมูลและคลังสินค้าและการประมวลผลข้อมูลโดยใช้กระบวนการและอัลกอริทึมทางสถิติและคณิตศาสตร์
ก่อนที่คุณจะเริ่มการขุดข้อมูลสิ่งที่คุณควรรู้
ก่อนที่ธุรกิจขนาดเล็กและ บริษัท ที่เพิ่งเริ่มต้นจะเข้ามากุมบังเหียนของการขุดข้อมูลพวกเขาจำเป็นต้องตรวจสอบให้แน่ใจว่าพวกเขาเข้าใจสิ่งที่เกี่ยวข้องและวิธีการใช้การขุดข้อมูลเพื่อให้ได้ประโยชน์สูงสุดสำหรับธุรกิจของพวกเขา ความรู้พื้นฐานเกี่ยวกับตลาดคู่แข่งและธุรกิจของคุณเป็นสิ่งสำคัญดังนั้นคุณจึงรู้ว่าควรกำหนดเป้าหมายอะไรในกลยุทธ์การขุดข้อมูลของคุณ การขุดข้อมูลสำหรับสตาร์ทอัพ
01
วิเคราะห์คู่แข่ง
รู้ว่าคุณอยู่ที่ใดในตลาดและเข้าใจว่าคู่แข่งของคุณทำการตลาดผลิตภัณฑ์และบริการอย่างไรและพวกเขาดึงดูดฐานลูกค้าร่วมกันของคุณอย่างไร
02
ใช้ข้อความเฉพาะ
ตรวจสอบให้แน่ใจว่าธุรกิจของคุณสามารถแก้ปัญหาให้กับลูกค้าได้และมีจุดยืนที่ชัดเจน
03
ยึดลูกค้าเป็นศูนย์กลาง
ธุรกิจในปัจจุบันจำเป็นต้องมอบประสบการณ์ของลูกค้าที่เกี่ยวข้องซึ่งให้ความสำคัญกับสิ่งที่ลูกค้าต้องการและจำเป็นตามพฤติกรรมการซื้อของพวกเขา
05
โอบกอดเทคโนโลยี
เริ่มใช้เทคโนโลยีที่ช่วยให้คุณสามารถใช้ประโยชน์จากข้อมูลและการวิเคราะห์เพื่อให้มีประสิทธิภาพประสิทธิผลได้รับข้อมูลและมอบประสบการณ์ที่ดียิ่งขึ้นให้กับลูกค้า
การขุดข้อมูลบางครั้งเรียกว่าการค้นพบความรู้และ บริษัท ที่เพิ่งเริ่มต้นและธุรกิจขนาดเล็กจำเป็นต้องได้รับความรู้ที่เหมาะสมซึ่งได้มาจากข้อมูลเพื่อให้สามารถแข่งขันได้และทำการตัดสินใจเชิงวิเคราะห์ที่ดีซึ่งจะช่วยให้ บริษัท ของพวกเขาเติบโตและเติบโต
เทคนิคการขุดข้อมูลที่แตกต่างกันสามารถให้คำตอบสำหรับคำถามทางธุรกิจที่เร่งด่วนที่สุดของคุณไม่ว่าจะเป็นเรื่องการตลาดการขายนิสัยของลูกค้าความเสี่ยงทางการตลาดโอกาสทางการเงินหรือการคาดการณ์ค่าใช้จ่ายในอนาคต
ประเภทของการขุดข้อมูล
เทคนิคการขุดข้อมูลหลัก ๆ มีสี่ประเภทที่ทั้งอุตสาหกรรมใช้:
วิธีนี้ดีที่สุดสำหรับกระบวนการขุดข้อมูลที่ง่ายมากและไม่ต้องอาศัยฟังก์ชันจากฐานข้อมูลหรือระบบคลังข้อมูล
สถาปัตยกรรมของการขุดข้อมูลแบบไม่มีการเชื่อมต่อ
สถาปัตยกรรมนี้ไม่ได้ใช้คุณสมบัติหรือฟังก์ชันที่นำเสนอโดยฐานข้อมูลหรือระบบคลังข้อมูลและดึงข้อมูลจากแหล่งที่มาเฉพาะและประมวลผลผ่านอัลกอริทึมที่แตกต่างกันเพื่อค้นหารูปแบบและจัดเก็บผลลัพธ์ในระบบไฟล์
กระบวนการขุดข้อมูลสำหรับการขุดข้อมูลแบบไม่มีการเชื่อมต่อ
เทคนิคนี้รวบรวมข้อมูลจากแหล่งที่มาเฉพาะและใช้อัลกอริทึมที่เหมาะสมในการประมวลผลข้อมูลนั้น
เทคนิคการขุดข้อมูลสำหรับการขุดข้อมูลแบบไม่มีการเชื่อมต่อ
ไม่มีการรวมเข้ากับฐานข้อมูลหรือคลังข้อมูล ไม่แนะนำให้ใช้การประมวลผลไฟล์แบบแบนเมื่อใช้ฐานข้อมูลหรือระบบคลังข้อมูล
ข้อดี
ข้อดี ได้แก่ การใช้อัลกอริธึมการขุดข้อมูลที่สำคัญและสามารถจัดเก็บผลลัพธ์ในระบบไฟล์
ข้อเสีย
ข้อเสียคือไม่ใช้ฐานข้อมูลหรือคลังข้อมูลที่มีประสิทธิภาพในการจัดระเบียบจัดเก็บและดึงข้อมูล ยังถือว่าเป็นสถาปัตยกรรมการขุดข้อมูลที่ค่อนข้างอ่อนแอ
การเชื่อมโยงในการขุดข้อมูลแบบไม่มีการเชื่อมต่อ
สามารถสร้างกฎการเชื่อมโยงที่แสดงสถานการณ์ค่าแอตทริบิวต์ที่มักเกิดขึ้นพร้อมกันในชุดข้อมูลใด ๆ
การจำแนกประเภทในการขุดข้อมูลแบบไม่มีการเชื่อมต่อ
ใช้แหล่งที่มาเช่นไฟล์แบบแบนเพื่อรวบรวมชุดข้อมูลเริ่มต้นสำหรับการขุดเนื่องจากฟังก์ชันคลังข้อมูลและระบบฐานข้อมูลไม่ได้นำมาใช้เป็นส่วนหนึ่งของขั้นตอนดังนั้นสถาปัตยกรรมนี้จึงแสดงถึงรูปแบบการออกแบบที่อ่อนแอในบางครั้ง
กรณีศึกษา – การขุดข้อมูลแบบไม่มีการเชื่อมต่อ
เทคนิคนี้ใช้เพื่อปรับปรุงแอพมือถือที่เน้นผู้ป่วยเป็นศูนย์กลางสำหรับการจัดการด้านสุขภาพ จุดประสงค์คือเพื่อใช้ผลลัพธ์สำหรับการติดตามและตรวจสอบผู้ป่วยการวินิจฉัยเบื้องต้นและการคำนวณความเสี่ยงและทำความเข้าใจวิธีจัดการกับความต้องการของผู้ป่วยให้ดีขึ้น การศึกษานี้ยังต้องการตรวจสอบว่าเทคนิคการขุดข้อมูลช่วยให้องค์ประกอบการจัดการตนเองของแอปด้านการดูแลสุขภาพเกี่ยวกับความแม่นยำและความน่าเชื่อถือได้อย่างไร
มีการผลิตบทความวิจัยที่แตกต่างกันสามสิบบทความซึ่งทำลายวิธีการขุดข้อมูลสำหรับการจัดการตนเองของผู้ป่วยผ่านแอพ การศึกษาสิบสามชิ้นพบระดับความแม่นยำ 90% หรือสูงกว่าและงานวิจัย 7 ชิ้นรายงานความแม่นยำมากกว่า 95% เกี่ยวกับประสิทธิภาพของอัลกอริทึมการขุดข้อมูล
เทคนิคการขุดข้อมูลกล่าวถึงความสามารถเช่นการคาดการณ์การประมาณและการตรวจจับสำหรับผู้ป่วยแต่ละรายในขณะที่รวบรวมข้อมูลของเขา / เธอ ผลลัพธ์เหล่านี้ช่วยในการพัฒนาแอปบนอุปกรณ์เคลื่อนที่อย่างต่อเนื่องซึ่งสามารถสร้างวิธีการใหม่ ๆ ในการดูแลสุขภาพแก่ผู้ที่มีภาวะเรื้อรัง
รูปแบบการขุดข้อมูลนี้รวบรวมส่วนของข้อมูลใด ๆภายในฐานข้อมูลที่มีความคล่องตัวและประสิทธิภาพมาก
สถาปัตยกรรมของ Loose Coupling Data Mining
ระบบขุดข้อมูลนี้ดึงข้อมูลจากภายในฐานข้อมูลและจัดเก็บผลลัพธ์และเป็นระบบที่ใช้หน่วยความจำ
กระบวนการขุดข้อมูล Loose Coupling
ระบบนี้ใช้ฟังก์ชันบางอย่างของระบบ DB และ DW ที่รวบรวมข้อมูลจากที่เก็บและดำเนินการขุดข้อมูลและจัดเก็บผลลัพธ์ในไฟล์ฐานข้อมูลหรือคลังข้อมูล
เทคนิคการขุดข้อมูลแบบ Loose Coupling
ระบบนี้ใช้ทั้งฐานข้อมูลและฟังก์ชันคลังข้อมูลเพื่อรวบรวมข้อมูลจากที่เก็บและดำเนินการขุดข้อมูลบนข้อมูลนั้น ผลลัพธ์จะถูกเก็บไว้ในไฟล์คลังข้อมูลหรือฐานข้อมูล
ข้อดี
ข้อดี ได้แก่ ข้อมูลแบบเรียลไทม์และเวลาแฝงต่ำความคล่องตัวที่เพิ่มขึ้นและความสามารถในการจ่ายเนื่องจากไม่จำเป็นต้องแปลข้อมูลให้เข้ากับท้องถิ่น
ข้อเสีย
ข้อเสียรวมถึงปัญหาที่อาจเกิดขึ้นกับความหมายภายในสคีมาและการตอบสนองการสืบค้นที่รวดเร็วน้อยลงเนื่องจากข้อมูลที่ไม่ได้แปลรวมทั้งการพึ่งพาแหล่งข้อมูล
การเชื่อมโยงในการขุดข้อมูลแบบ Loose Coupling
ควรสร้างกฎการเชื่อมโยงที่แสดงค่าความหมายในอินพุตแบบสอบถามที่นำมาจากฐานข้อมูลต้นทางไปรวมกับชุดผลลัพธ์
สิ่งนี้ทำให้ระบบการขุดข้อมูลสามารถจำแนกตามประเภทของฐานข้อมูลที่ขุดได้ประเภทของความรู้ที่ขุดได้และวิธีการหรือแอปพลิเคชันที่ถูกนำไปใช้
กรณีศึกษา – Loose Coupling Data Mining
เทคนิคนี้ใช้ในการดำเนินการสองโมเดลในคลัสเตอร์โลจิสติกที่แตกต่างกันสำหรับการพึ่งพาเวิร์กโฟลว์และกำหนดเวลาในอุตสาหกรรม Telco ลูกค้าถูกแบ่งออกเป็นหลายคลัสเตอร์ที่มีการจัดกลุ่มพฤติกรรมคล้ายกันและข้อมูลคลาสจะถูกประมวลผลโดยการกรอง 39 คอลัมน์และ 300 ล้านแถวของข้อมูลดิบออกเป็น 20 คอลัมน์ซึ่งรวมคุณลักษณะของลูกค้าค่าบริการการสื่อสารและข้อมูลข้อความ
บริษัท สามารถได้รับระดับความแม่นยำ 89% เมื่อเทียบกับผลลัพธ์จริงและผู้ให้บริการโทรคมนาคมสามารถปรับปรุงประสบการณ์ให้กับลูกค้าได้
คุณอาจต้องการระบุข้อมูลพื้นฐานหรือความรู้เกี่ยวกับโดเมนในส่วนของข้อมูลที่กำลังจะถูกขุดเนื่องจากสามารถช่วยในกระบวนการค้นหาความรู้และการระบุรูปแบบภายในข้อมูลและสามารถช่วยแนะนำกระบวนการได้
สถาปัตยกรรมของการขุดข้อมูลแบบ Semi-Tight Coupling
ระบบนี้ใช้ข้อมูลทะเลสาบหรือส่วนประกอบของระบบคลังข้อมูลที่แตกต่างกันสำหรับกิจกรรมการขุดข้อมูลเช่นการจัดทำดัชนีการเรียงลำดับและการรวมและผลลัพธ์บางอย่างสามารถเก็บไว้ในฐานข้อมูลหรือคลังข้อมูลเพื่อประสิทธิภาพที่ดีขึ้น
กระบวนการขุดข้อมูลการเชื่อมต่อแบบกึ่งแน่น
กระบวนการนี้เชื่อมโยงระบบเหมืองข้อมูลกับคลังข้อมูลหรือฐานข้อมูลและสามารถใช้ข้อมูลพื้นฐานบางอย่างกับฐานข้อมูลได้
เทคนิคการขุดข้อมูลการเชื่อมต่อแบบกึ่งแน่น
ระบบนี้สามารถเชื่อมต่อกับระบบคลังข้อมูลซึ่งสามารถประยุกต์ใช้พื้นฐานการขุดข้อมูลบางอย่างในฐานข้อมูลเชิงสัมพันธ์ / ระบบคลังข้อมูล ข้อมูลพื้นฐานอาจรวมถึงการจัดทำดัชนีการเรียงลำดับฮิสโตแกรมการรวมและการคำนวณล่วงหน้าของวิธีการทางสถิติที่แตกต่างกัน
ข้อดีและข้อเสียของการขุดข้อมูลแบบ Semi-Tight Coupling
ข้อดี ได้แก่ ความสามารถในการเชื่อมโยงไปยังฐานข้อมูลหรือระบบคลังสินค้าโดยใช้คุณสมบัติต่างๆของคลังข้อมูลและระบบฐานข้อมูลสำหรับวิธีการขุดข้อมูลเช่นการเรียงลำดับหรือการจัดทำดัชนี นอกจากนี้ผลลัพธ์ยังสามารถเก็บไว้ในฐานข้อมูลหรือคลังข้อมูลเพื่อประสิทธิภาพที่ดีขึ้น
การเชื่อมโยงในการขุดข้อมูลการเชื่อมต่อแบบกึ่งแน่น
มาตรการสำหรับกฎการเชื่อมโยงรวมถึงองค์ประกอบที่สนับสนุนเช่นเปอร์เซ็นต์ของงานขุดข้อมูลที่รูปแบบกฎปรากฏขึ้นและความมั่นใจในความแข็งแกร่งที่วัดได้ของผลกระทบของกฎ
การจำแนกประเภทในการขุดข้อมูลการเชื่อมต่อแบบกึ่งแน่น
การจำแนกประเภทในการขุดข้อมูลของสถาปัตยกรรมนี้รวมถึงฟังก์ชันฐานข้อมูลเชิงสัมพันธ์และคลาสย่อยที่กำหนดโดยคุณลักษณะและรูปแบบจิตใจ
การขุดข้อมูลการเชื่อมต่อแบบกึ่งแน่น
กรณีศึกษา – การขุดข้อมูลการเชื่อมต่อแบบกึ่งแน่น
เทคนิคนี้ถูกนำไปใช้ในกรณีศึกษาด้านการดูแลสุขภาพเพื่อประเมินว่าการเข้ารับการรักษาโดยไม่อดทนส่งผลต่อผู้ป่วย Medicare และ Medicaid ตามภูมิภาครวมถึงระยะเวลาในการเยี่ยมโดยเฉลี่ยและผลกระทบทางการเงินที่เกี่ยวข้อง ข้อมูลประชากรของผู้ป่วยได้รับการพิจารณาในการวิเคราะห์และใช้รายงานเพื่อทำความเข้าใจให้ดีขึ้นว่าอายุมีผลต่อการดูแลและการสูญเสียทางการเงินต่อสถาบันอย่างไร
ขั้นแรกต้องดึงข้อมูลต้นทางโดยการคำนวณแบบสอบถามและสร้างมุมมองของแหล่งที่มาที่มีข้อมูลที่จะถูกประมวลผล จากนั้นจำนวนกลุ่มภายในข้อมูลต้นทางจะต้องถูกนำมาพิจารณา
การขุดข้อมูลการเชื่อมต่อแบบแน่น
01
ชั้นข้อมูลทำหน้าที่เป็นอินเทอร์เฟซสำหรับแหล่งข้อมูลที่แตกต่างกันและผลลัพธ์ที่ขุดได้จะถูกเก็บไว้ในเลเยอร์นี้
02
แอปพลิเคชันการขุดข้อมูลเลเยอร์นี้ดึงข้อมูลจากฐานข้อมูลและงานการแปลงจะดำเนินการที่นี่เพื่อปรับเปลี่ยนข้อมูลให้อยู่ในรูปแบบที่จำเป็นโดยข้อมูลจะถูกประมวลผลโดยใช้อัลกอริธึมการขุดข้อมูลที่เหมาะสม
03
Front-end layer ให้อินเทอร์เฟซที่ใช้งานง่ายสำหรับผู้ใช้ที่อำนวยความสะดวกในการโต้ตอบกับระบบและผลลัพธ์สามารถมองเห็นได้ในรูปแบบในเลเยอร์ส่วนหน้า
กระบวนการขุดข้อมูลการเชื่อมต่อแบบแน่น
ที่นี่ฐานข้อมูลหรือคลังข้อมูลทำหน้าที่เป็นคุณลักษณะการดึงข้อมูลสำหรับการขุดข้อมูลโดยใช้การรวมและดำเนินกิจกรรมการขุดข้อมูล สถาปัตยกรรมมีความสามารถในการปรับขยายประสิทธิภาพที่เพิ่มขึ้นและข้อมูลในตัว
เทคนิคการขุดข้อมูลการเชื่อมต่อแบบแน่น
การรวมระบบเหมืองข้อมูลเข้ากับคลังข้อมูลหรือฐานข้อมูลอย่างราบรื่นเกิดขึ้นในเทคนิคนี้ ระบบย่อยทำหน้าที่เป็นองค์ประกอบเดียวของระบบสารสนเทศ
ทั้งนี้บริษัทเคแอนด์โอ จึงได้มุ่งเน้นการจัดการแก้ไขปัญหา จัดการเอกสาร ด้านเอกสารขององค์กรมาอย่างยาวนาน และ ให้ความสำคัญกับด้านงานเอกสาร ต่อลูกค้าเป็นอย่างดี จนถึงปัจจุบันก็ได้ความยอมรับจากองค์กร ขนาดใหญ่ ขนาดกลาง และขนาดเล็กมากมาย จึงใคร่ขออาสาดูและปัญหาด้านเอกสารให้กับองค์กรของท่านอย่างสุดความสามารถ เพราะเราเป็นหนึ่งในธุรกิจ ระบบจัดเก็บเอกสาร ที่ท่านไว้ใจได้
สนใจรับคำปรึกษาด้านวางระบบจัดการเอกสารอิเล็กทรอนิกส์ EDMS โดยทีมงานผู้เชี่ยวชาญจาก K&O ที่มีประสบการณ์มากว่า 15 ปี รวมถึงซอฟต์แวร์ระดับโลก ติดต่อ 0 2 – 8 6 0 – 6 6 5 9
ส า ม า ร ถ รั บ ช ม วี ดี โ อ ส า ธิ ต วิ ธี ก า ร ใช้ ง า น จ ริ ง ไ ด้ ที่ นี่
ความแตกต่างระหว่างการทำเหมืองข้อมูลและคลังข้อมูล
ให้เราตรวจสอบความแตกต่างระหว่าง data mining และ data data ด้วยความช่วยเหลือของกราฟเปรียบเทียบที่แสดงด้านล่าง
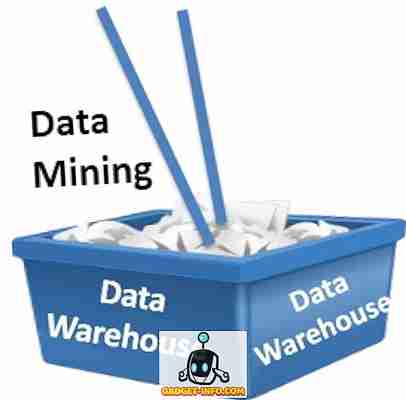
การขุดข้อมูลเป็นกระบวนการ ค้นพบความรู้ ซึ่งคุณ ไม่เคยคาดหวังว่า จะ มีอยู่ในฐานข้อมูลของคุณ การใช้เครื่องมือ Query แบบดั้งเดิมคุณสามารถดึงข้อมูลที่ทราบจากข้อมูลเท่านั้น แต่ Data mining เป็นวิธีการในการ ดึงข้อมูลที่ซ่อนอยู่ออกจากข้อมูล การขุดข้อมูลจะดึงข้อมูลที่มีความหมายจากฐานข้อมูลที่สามารถใช้สำหรับ การตัดสินใจ
การค้นพบความรู้ในฐานข้อมูลที่เรียกว่า KDD จะ แสดง ความสัมพันธ์ และ รูปแบบ ความสัมพันธ์อาจอยู่ระหว่างวัตถุสองชิ้นหรือมากกว่านั้นระหว่างคุณสมบัติของวัตถุเดียวกัน แพทเทิร์นเป็นผลมาจากการขุดข้อมูลที่แสดงลำดับของข้อมูลที่เข้าใจได้ง่ายซึ่งช่วยในการตัดสินใจ
ขั้นตอนที่เกี่ยวข้องใน KDD คือการค้นพบความรู้ในฐานข้อมูลสามารถสรุปได้เป็นครั้งแรกการ เลือก ชุดข้อมูลที่จะต้องดำเนินการขุดข้อมูล ถัดไปคือ การประมวลผลล่วงหน้า ที่เกี่ยวข้องกับการลบข้อมูลที่ไม่สอดคล้องกัน จากนั้นการ แปลงข้อมูล ที่ซึ่งข้อมูลถูกแปลงเป็นรูปแบบที่เหมาะสมสำหรับการขุดข้อมูล ถัดไปคือ data mining ขั้นตอนวิธี data mining นำไปใช้กับข้อมูล ในที่สุด การตีความและการประเมินผล ที่เกี่ยวข้องกับการแยกความสัมพันธ์หรือรูปแบบระหว่างข้อมูล
การขุดข้อมูลนั้นเหมาะสมในสภาพแวดล้อมคลังข้อมูลที่เก็บข้อมูลในลักษณะรวมและสรุป มันกลายเป็นเรื่องง่ายที่จะขุดข้อมูลในคลังข้อมูล
Data Warehouse เป็นตำแหน่งศูนย์กลางที่ข้อมูลที่ รวบรวมจากหลายแหล่งจะถูกเก็บไว้ภายใต้ schema แบบรวมศูนย์เดียว การรวบรวมข้อมูลเริ่มต้นแหล่งต่าง ๆ ขององค์กรจากนั้นทำความสะอาดและแปลงและจัดเก็บในคลังข้อมูล เมื่อมีการป้อนข้อมูลในคลังข้อมูลข้อมูลนั้นจะอยู่ที่นั่นเป็นเวลานานและสามารถเข้าถึงได้เกินเวลา
Data Warehouse เป็นการผสมผสานกันอย่างลงตัวของเทคโนโลยีเช่น การสร้างแบบจำลองข้อมูลการเก็บข้อมูลการจัดการข้อมูลการจัดการข้อมูลเมตาการจัดการร้านเครื่องมือในการพัฒนา เทคโนโลยีทั้งหมดเหล่านี้รองรับฟังก์ชั่นเช่นการ แยกข้อมูลการแปลงข้อมูลการจัดเก็บข้อมูลให้ส่วนต่อประสานผู้ใช้สำหรับการเข้าถึงข้อมูล
คลังข้อมูลไม่ใช่ผลิตภัณฑ์หรือซอฟต์แวร์ แต่เป็นสภาพแวดล้อมข้อมูลที่ให้ข้อมูลเช่นมุมมองแบบรวมขององค์กร คุณสามารถเข้าถึงข้อมูลปัจจุบันและข้อมูลย้อนหลังขององค์กรซึ่งช่วยในการตัดสินใจ สนับสนุนธุรกรรมที่ทำเพื่อการตัดสินใจโดยไม่กระทบต่อระบบปฏิบัติการ มันเป็นทรัพยากรที่มีความยืดหยุ่นในการรับข้อมูลเชิงกลยุทธ์
ความแตกต่างที่สำคัญระหว่างการทำเหมืองข้อมูลและคลังข้อมูล
• มีความแตกต่างพื้นฐานที่แยก data mining และ data warehousing ที่ data mining เป็นกระบวนการของการแยกข้อมูลที่มีความหมายจากฐานข้อมูลขนาดใหญ่หรือคลังข้อมูล อย่างไรก็ตามคลังข้อมูลจัดให้มีสภาพแวดล้อมที่ข้อมูลถูกเก็บไว้ในรูปแบบบูรณาการซึ่งช่วยให้การขุดข้อมูลง่ายขึ้นเพื่อดึงข้อมูลได้อย่างมีประสิทธิภาพมากขึ้น
การขุดข้อมูลสามารถทำได้ก็ต่อเมื่อมีฐานข้อมูลขนาดใหญ่ที่รวมอยู่ในตัวเช่นคลังข้อมูล ดังนั้นคลังข้อมูลจะต้องเสร็จสิ้นก่อนทำการขุดข้อมูล คลังข้อมูลจะต้องมีข้อมูลในรูปแบบที่รวมเข้าด้วยกันเพื่อให้การขุดข้อมูลสามารถดึงความรู้ออกมาได้อย่างมีประสิทธิภาพ